First studies with Quantum Machine Learning at CERN’s LHCb experiment
The Department of Physics and Astronomy G. Galilei and INFN-Padova division have taken a step further in the application of Quantum Algorithms to the analysis of particle physics data.
In fact, the LHCb collaboration at the CERN laboratory, which sees the participation of both the Department of Physics and the INFN section, has just published a forward-looking research paper exploiting, for the first time, Quantum Machine Learning techniques for the identification of the b-quark charge at the LHC. This is a complex task since b-quarks produce sprays of particles called jets, and the information on their charge is diluted in a large number of decay products. Nevertheless it is important to solve it in order to perform many important measurements.
The paper just appears on the Journal of High Energy Physics (JHEP). Further information is available online on the https://link.springer.com/article/10.1007/JHEP08%282022%29014
“Given the rapid progress of quantum computers and quantum technologies, it is natural to start investigating if and how quantum algorithms can be executed on such new hardware”, Donatella Lucchesi, professor at the Department of Physics and Astronomy, said, “and whether the LHCb particle physics use-cases can benefit from the new technology and paradigm”.
“Although it has already been used to solve few particle physics problems, this is the first time that Quantum Machine Learning is used by a LHC experiment to tackle a complex task”, Lorenzo Sestini, researcher of the INFN section, comments, “LHCb applied for the first time these news algorithms to the task of the hadronic jet charge identification, by using a detailed simulation that faithfully reproduce the data. The algorithm is now ready to run on the real data collected by the experiment”.
The algorithm designed for the ‘Quantum Machine Learning for b-jet charge identification’ study has been thought for running on a quantum computer. The first tests have been conducted on realistic quantum simulators, due to the limited availability of hardware, but now the tests of the algorithm are running on real quantum computers.
As demonstrated in the paper, the Quantum Machine Learning method reaches optimal performance with a lower number of events with respect to other classical (non-quantum) and modern types of artificial intelligence like Deep Neural Networks, which helps in reducing the computing resources’ usage, which will become a key point with the huge amount of data collected at LHCb in future years. Moreover quantum computers could in principle help us in reducing the power consumption for this kind of heavy-computing tasks. “It is great to merge together two distinct research fields such as High Energy Physics and Quantum Computing to reach a common task“, says Davide Zuliani, a PhD student of Padua Physics PhD school, “and it has been definitely an exciting experience study these brand new algorithms: we started with the graduation thesis of Davide Nicotra, now PhD student in Maastricht, and in few months we discovered surprising potentialities. This new research field is very promising and, given the huge impact that this new technology might have, we are working together with a lot of people to understand how to effectively exploit Quantum Computing to improve the discovery horizon of the experiments at the LHC“.
Exploitation of Quantum Machine Learning in particle physics experiments is still in its infancy. As physicists gain experience with Quantum Computing, drastic improvements in hardware and computing technology are expected given the worldwide interest and investment in Quantum Computing.
Read more https://link.springer.com/article/10.1007/JHEP08%282022%29014
Contacts
Donatella Lucchesi – Department of Physics and Astronomy – University of Padua
Lorenzo Sestini – INFN Padua Division
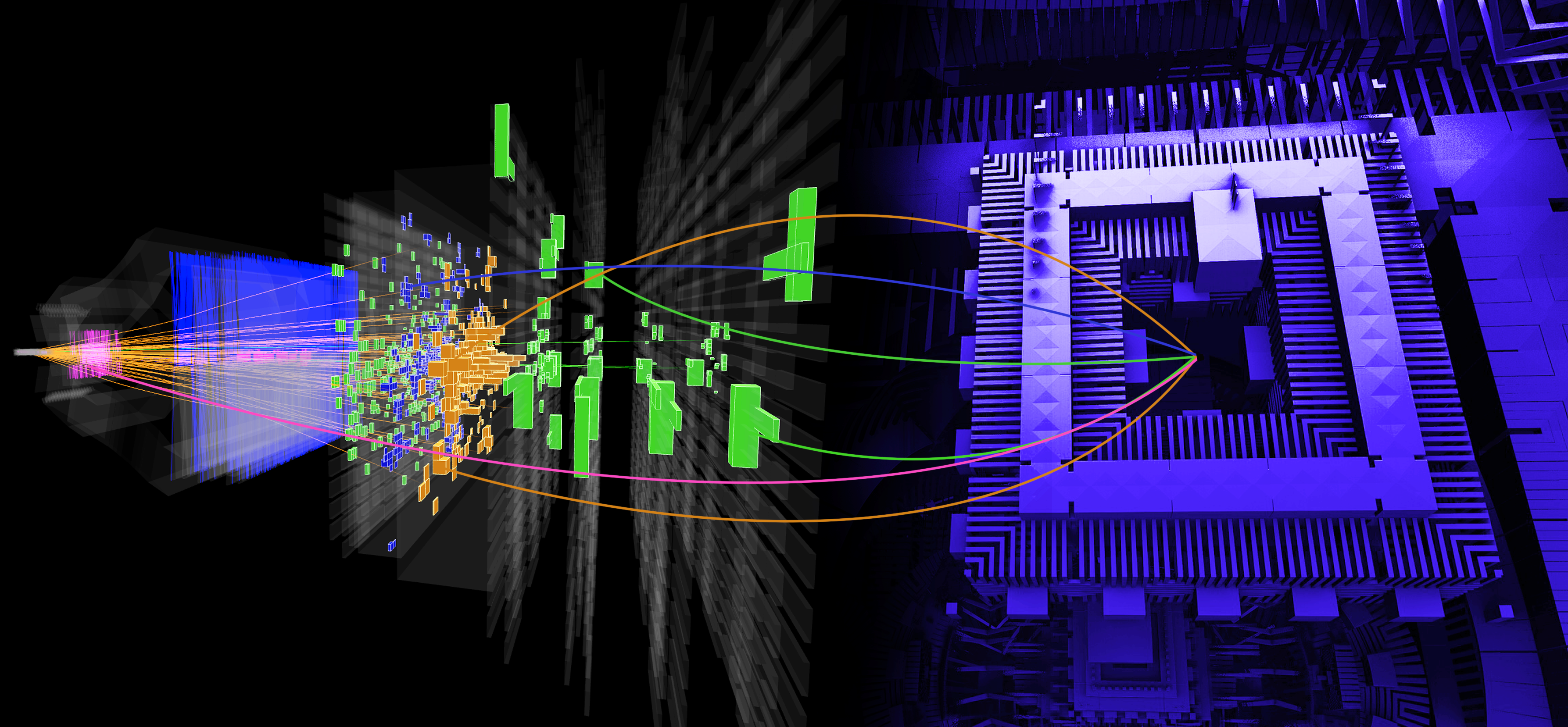